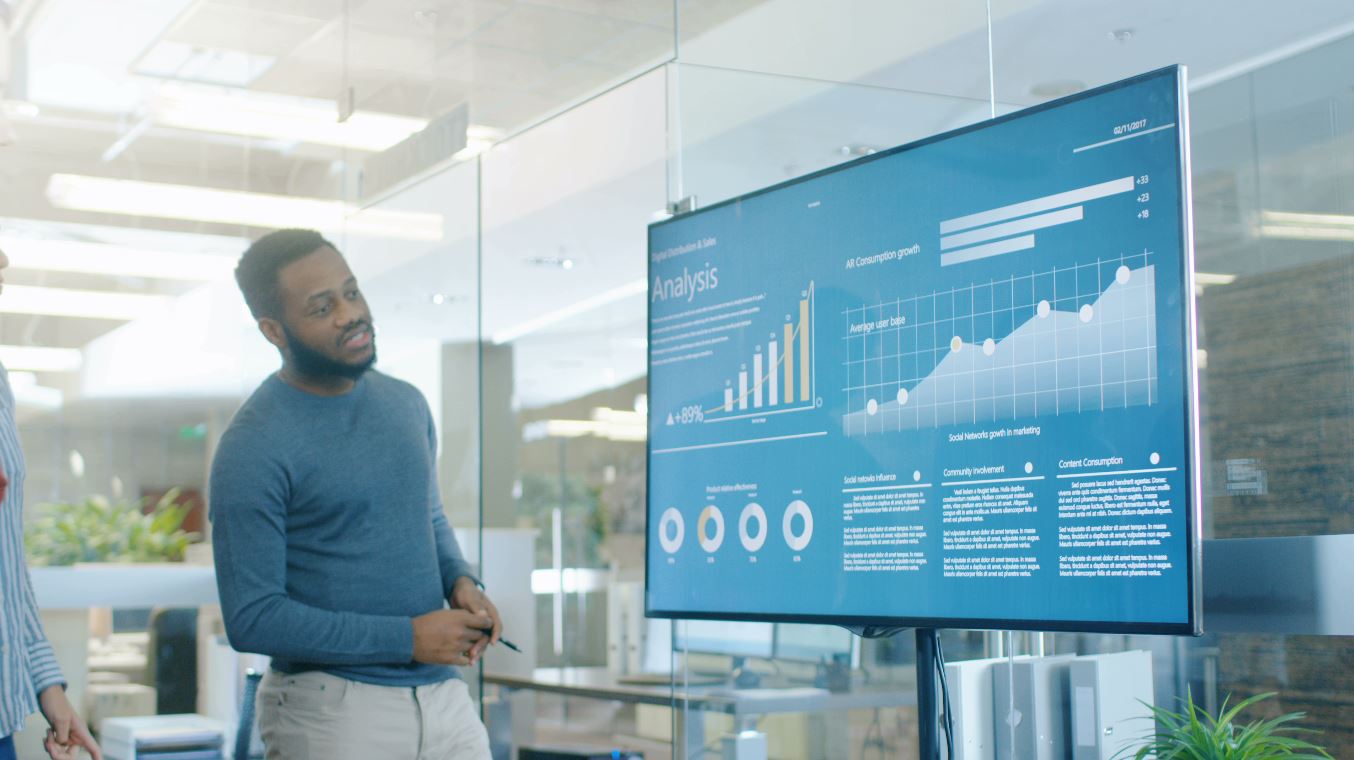
03 Aug 2019 Introduction to reliability and lifetime analysis of electronic systems and devices
This article describes the statistical reliability and lifetime analysis with the focus on electronic devices. It contents the basics of statistical methods. These include lifetime distributions for electronic components, hypothesis tests and quality of data characteristics. Practical data analysis is also part of this paper. In summary, you will find some recommendations and tricks for statistical analysis.
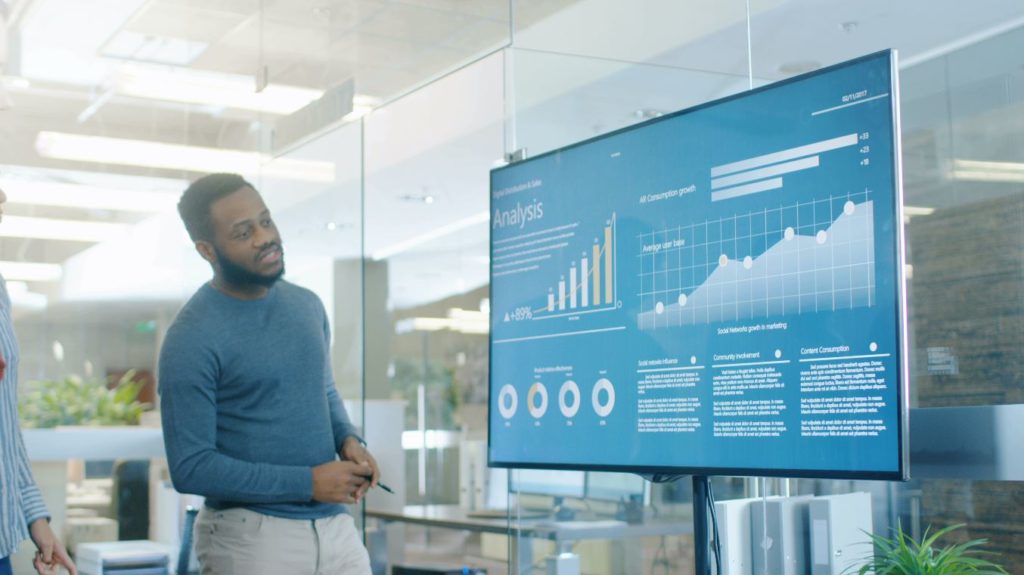
Content
- From the representative sample to the predicted behavior of all products
- Lifetime distributions: Describe the deviation of the service life through formulas
- Hypothesis: Should you complain to your supplier?
- Data characteristics: From ok/not ok to physical measured values
- Practical data analysis: Graphics first, p-values second
- Tips and tricks for analysis
From the representative sample to the predicted behavior of all products
The aim of every statistical analysis is to be able to deduce the behavior of all products (population) from a small number of test samples. For this, it is necessary that the sample is representative. This means that the sample must reflect all characteristics and weights of the population.
Example: To determine the average lifetime of a capacitor, the sample must include all capacitor types, manufacturers, sizes, styles, etc. that are intended to be used. If only one type is tested, the sample is not representative of the population of all capacitor types.
Whenever a sample is used to draw conclusions about the population, statistical distribution models or tests are utilized.
Lifetime distributions: Describe the deviation of the lifetime through formulas
During the production of components, products, etc. there are variances in the electrical, physical or geometric properties of the used material. If a characteristic (e.g. the capacity) of all capacitors is tested, resulting values lie within certain limits and accumulate at specific results.
The mathematical description of this accumulation is called distribution. The best-known distribution is the Gaussian or bell curve, also known as the normal distribution. The normal distribution is defined by the mean value and the standard deviation. Video 1 shows a distribution with different standard deviations.
Video 1: Test for Normal distribution , source youtube
The Weibull distribution is often used for lifetime data. Whereas the normal distribution is symmetrical, the Weibull distribution is usually skewed. By varying your parameters, the Weibull distribution is very flexible. It is described by a form and scale parameter.
Hypothesis: Should you complain to your supplier?
The purpose of hypothesis testing is to make a statement about the population, based on one sample. Example: Your supplier promises that an electrical resistor will work for 1,000 operating hours.
Null hypothesis H0: The statement of your supplier is correct
Alternative hypothesis H1: The statement of your supplier is incorrect. You want to avoid two types of errors:
- The statement of your supplier is correct. However, a test leads you to the conclusion that his statement is incorrect and you accuse him of being wrong.
- Your supplier’s statement is incorrect. However, your lifetime test shows that everything is in order.
To avoid these errors, an appropriate sample quantity is required. You can also use the alpha level to determine your personal risk tolerance. Example: When testing an electrical device, the result can only be functioning or not functioning. The probability for good to bad is 50:50.
- Testing the first device: not functioning.; probability: 50%
- Testing the second device: not functioning; probability of two successive components being defective: 50% * 50% = 25%
- Testing another device: not functioning; probability of three successive components being defective: 50% * 50% * 50% = 12,5%
An alpha level of 0.05 indicates that your risk tolerance is 5 %, i.e. once more than five of 100 defective parts have been found, it cannot be trusted that the supplier is reliable.
In order to avoid the second type of error, selectivity tests are performed. You specify how sure you want to be that this error will not occur. A higher level of safety requires a larger sample size, respectively a smaller dispersion of the test objects (for example deviation in normal distribution).
Data characteristics: From okay/not okay to physical measured values
The following generally applies to all tests and their respective statistical analyses: The more information, the better. The goal is always numerical values, meaning continuous quantitative characteristics. The following list is sorted according to increasing data quality:
- Qualitative characteristics
- Good/Bad
- Okay/not okay
- Ordinal (regulatory relationship)
- Bigger / smaller than…
- School grades
- Quantitative characteristics: Measured values
It is crucial to always use raw data, not previously averaged values, for the analysis.
Practical data analysis: Graphics first, p-values second
When performing statistical analysis, always get an overview with graphics first. Typical graphs that deliver a good first overview are:
- Histograms or point diagrams
- For optical first visual impression of the data distribution
- Are there several mountains and valleys?
- Are there outliers?
- Is the distribution skewed or symmetrical?
- Is the distribution right- or left-aligned?
- Time series diagrams
- Are there temporal effects?
- Is the data drifting?
- Are there outliers?
- Box-Plots
- Are there significant differences between two groups?
- How strong is the deviation within one group?
- Are there outliers?
- Scatter plots
- Are there correlations between two parameters?
After gaining an overview, with the next step is descriptive statistics. This includes the calculation of mean value, median and standard deviation and is usually followed by distribution identifications and hypothesis tests.
Tips and tricks for analysis
- Definition of the goal data to be measured:
- What is the aim of the test?
- What load should the lifetime test accurately reflect?
- What will be measured?
- How will it be measured?
- What is the end-of-life criteria?
- Is the testing to be carried out until the end of the product’s lifetime?
- 2. Collecting knowledge, assumptions, and questions about the relationship between test parameters and lifetime. Examples are:
- An increase of 10 K test temperature reduces the lifetime by one order of magnitude.
- The ∆T has the greatest influence
- How do holding times influence the result?
- 3. Planning the tests: Number of test items, test duration, different test points;
Statistical design of experiments and test plans can be used for this purpose. - Can all questions be answered through the tests?
- Testing: Check as early as possible whether the tests fulfils its purpose. Avoid protracted series of tests that do not lead to the desired result.
- Test evaluation: One picture says more than 1,000 words – always start with a visual analysis. Write down your interpretation and assumption for each graph.
- Use statistical tests to check whether you can confirm these interpretations and assumptions.
- Verify your results by means of another test.
Video 2: Explanation of power and sample size:, sourceYoutube
Interested in further information? Contact us!